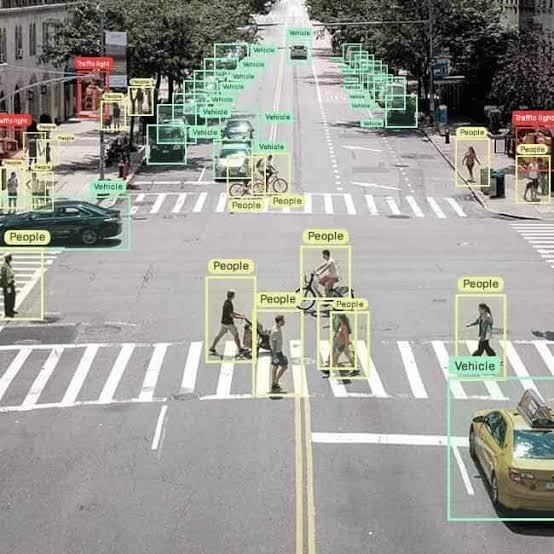
In the rapidly evolving fields of machine learning, artificial intelligence, and data analysis, the importance of high-quality annotated data cannot be overstated. Data annotation, the process of labelling or tagging data for training machine learning models, plays a crucial role in enabling accurate predictions and reliable insights.
However, data annotation is not without its challenges. In this blog post, we will explore the common challenges faced during the data annotation process in the United States and provide strategies on how to overcome them.
We will delve into topics such as lack of standardization, cost and scalability, annotator bias, privacy and data security, quality control, and technological limitations.
I. Lack of Standardization and Consistency
One of the significant challenges in data annotation is the lack of standardized guidelines, which can lead to inconsistent annotations and compromised data quality.
To overcome this challenge, it is crucial to establish annotation guidelines specific to the United States. These guidelines should define annotation conventions, labelling criteria, and annotation methodologies.
Organizations offering data annotation services, such as “data annotation service,” can play a vital role in creating and implementing standardized annotation practices.
By partnering with these services, businesses can ensure consistent and high-quality annotated data, enhancing the effectiveness of their machine learning models.
II. Cost and Scalability
Data annotation can be a resource-intensive process, both in terms of time and finances. Scaling annotation efforts to handle large datasets within limited budgets poses a significant challenge.
To address this, businesses can explore cost-effective strategies, such as crowdsourcing or leveraging annotation platforms. Data annotation service providers can offer scalable solutions by tapping into a global pool of annotators and optimizing the annotation workflow.
By leveraging these cost-saving approaches, organizations can manage their data annotation expenses efficiently and achieve scalability without compromising on quality.
III. Annotator Bias and Subjectivity
Annotator bias and subjectivity can introduce inherent limitations in data annotation, impacting the performance and fairness of machine learning models. Overcoming these challenges requires the establishment of diverse annotator teams and providing comprehensive training on bias mitigation.
By involving annotators from various backgrounds and perspectives, organizations can reduce bias and enhance the representativeness of annotated data.
It is crucial to partner with “data annotation service” providers that prioritize diversity and offer rigorous training programs to their annotators. Through such collaborations, businesses can ensure unbiased and objective annotations that improve the robustness of their machine learning models.
IV. Privacy and Data Security
In the era of heightened data privacy concerns, protecting sensitive information during data annotation is paramount. This challenge is particularly relevant in the United States, given its stringent data protection regulations.
Organizations must carefully evaluate the data annotation service providers they engage with to ensure compliance with privacy laws and maintain data security. An emphasis on secure data transfer, encrypted communication channels, and strict confidentiality agreements is essential.
Businesses should partner with “data annotation service” providers that prioritize privacy and offer robust data security measures, safeguarding their valuable datasets.
V. Quality Control and Annotation Validation
Maintaining high-quality standards throughout the data annotation process is crucial to ensure accurate and reliable machine learning models. However, achieving consistent quality control can be challenging.
To address this, organizations should implement robust quality control measures, including double-checking annotations, conducting regular audits, and establishing feedback loops with annotators.
Additionally, incorporating an annotation validation phase, where a subset of annotated data is independently reviewed by experts, can help verify the accuracy and reliability of annotations.
By partnering with “data annotation service” providers that prioritize quality control and offer rigorous validation processes, businesses can enhance the credibility of their annotated datasets.
VI. Technological Limitations
Technological limitations in data annotation can range from complex annotation requirements to inadequate annotation tools.
Overcoming these challenges requires staying up-to-date with the latest advancements in annotation technology. Image annotation is a specific area that has seen significant technological progress, with innovations such as bounding box annotation, semantic segmentation, and instance segmentation. By leveraging state-of-the-art annotation tools and partnering with “data annotation service” providers that embrace technological advancements, businesses can overcome these limitations and benefit from more efficient and accurate annotations.
Conclusion
Data annotation is a critical component of building robust machine learning models and extracting meaningful insights. While challenges in data annotation are inevitable, proactive strategies can help overcome them effectively. By addressing the lack of standardization, managing costs, mitigating annotator bias, ensuring privacy and data security, implementing quality control measures, and leveraging technological advancements, businesses in the United States can navigate the data annotation landscape successfully. Partnering with reliable “data annotation service” providers can significantly contribute to overcoming these challenges, enabling organizations to obtain high-quality annotated data for their machine learning projects and fuelling advancements in various industries.